Financial Firms Explore ChatGPT and Generative AI
July 17, 2023 | By: Ivy Schmerken
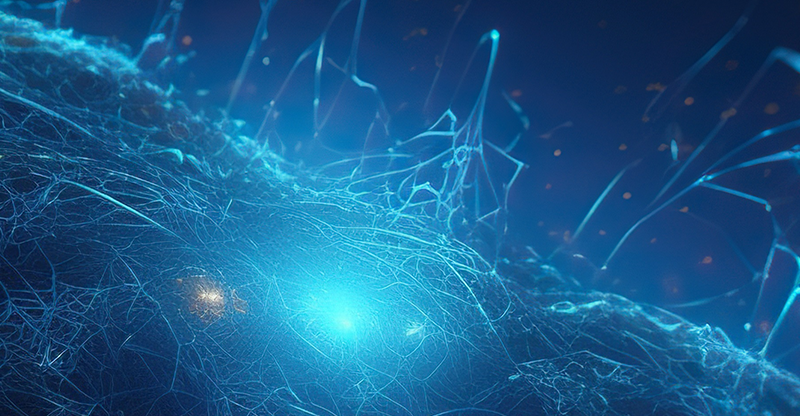
With the frenzy around OpenAI’s ChatGPT technology and generative artificial intelligence trained on vast collections of unstructured data to create large language models, there’s a race to experiment with these new tools in capital markets.
The release of ChatGPT by OpenAI last year, and its viral success in responding to human prompts in natural language, has ignited interest in generative AI technology, and has pushed asset managers and trading firms to grasp the technology rather than risk falling behind.
Wall Street firms have used artificial intelligence and machine learning for years, but ChatGPT has shown that generative AI systems, like ChatGPT and Google’s Bard, among others, such as AI startup Anthropic, can create humanlike conversation, text, images, and computer code.
Despite concerns about ChatGPT’s tendency to confidently provide false information not supported by the training data, (known as hallucination), financial firms and fintech providers are experimenting with specific use cases for generative AI using natural language processing to then create large language models (LLMs) as a result of the training.
ChatGPT’s ability to rapidly summarize reports, filter, sort and rank massive quantities of information could boost productivity for traders, analysts and other finance professionals, those familiar with the technology said.
In May, Bloomberg reported that generative AI is being used “to speed up the grunt work” or menial, repetitive tasks given to junior employees at hedge funds – “from reviewing market research to writing basic code and summarizing fund performance.”
Speaking on the May 4 Wall Street Horizon’s Data Minds webinar, Nick Colas, Founder of DataTrek Research, who writes a financial markets newsletter, said that when it comes to basic queries about the market, “(Google’s) Bard is better than Chat GPT which cuts off at 2021. “So, it’s not even useful for analyzing last year, and it will just give you a wrong answer.”
There are limitations to ChatGPT ‘s understanding. “ChatGPT doesn’t have any sentient understanding of what you are sending it. It’s just using statistics to predict what is most likely the next word. It’s not like a general artificial intelligence that is able to produce novel ideas or solve complex problems independently,” said Andy Mahoney, Managing Director of FlexTrade EMEA.
Many predict that generative AI will serve as an assistant performing repetitive tasks so that humans can focus on higher-level work. “As we talk about the future of AI, it’s looking at efficiency and automated insights and then potentially freeing up resources for higher valued or higher skilled work,” said Kelly Fryer, Executive Director of FinTech Sandbox, a non-profit that supports early-stage companies in fintech.
“While there is a big fear that AI will take over someone’s job, I think of [AI] as adding value– or as making [tools] for getting more specific portfolio construction or looking at more effective comparisons across different industries or across companies you are looking at on an apples-to-apples basis,” said Fryer.
Though Chat GPT is not perfect, this has not stopped some of the largest wealth management firms from exploring generative AI by training LLMs on the vast amounts of data they hold.
JPMorgan Chase is developing a ChatGPT-like software service based on artificial intelligence to select investments for customers, reported CNBC in May. The company applied to trademark the product IndexGPT, according to the filing. The filing mentions “cloud computing software using artificial intelligence” for “software analyzing and selecting securities tailored to customer needs.”
CNBC also reported that Morgan Stanley is rolling out an advanced chat box powered by OpenAI’s latest technology. In development for the past year, the AI tool is being tested with 300 financial advisors and will be released in the next few months. The tool is designed to help the bank’s 16,000 advisors “tap the bank’s enormous repository of research and data.”
“The promise of why so many are excited about large language models is that financial institutions are sitting on troves of data and don’t know how to extract insights or get value out of it,” said Jess Stauth, Chief Investment Officer, Systematic Equity at Fidelity Investments, who spoke on the Data Minds webinar in May hosted by Wall Street Horizon.
LLMs for Finance
In March, Bloomberg announced the development of BloombergGPT, a new large scale generative AI model which has been specifically trained on a wide range of financial data to support a diverse range of tasks in natural language processing. While ChatGPT 3.0 is trained on data available on the Internet, BloombergGPT is trained on the firm’s extensive proprietary data – “an archive of 363 billion English-language financial tokens supplemented with a 345 billion token public data set, resulting in a training corpus exceeding 700 billion tokens” – for the specific domain of financial services.
On the Data Minds webinar hosted by Wall Street Horizon, Rich Brown, the former head of data management for Citadel, said he was excited about ChatGPT’s impact on analyst productivity. But Brown and other panelists noted that ChatGPT is trained on data from the Internet through 2021.
“When data is on the Internet, analysts still need to worry about the accuracy and whether it’s fake,” said Brown. “With the introduction of Bloomberg’s LLM, the context and domain are very narrowly defined and will have important data sets, earnings information, conference call transcripts, 10Ks and 10Qs, he said.
According to private equity firm Andreessen Horowitz’s blog, financial services companies sitting on vast quantities of finance data, can use this data to fine-tune LLMss (or train them from scratch, like Bloomberg GPT). “They will be able to quickly produce answers to almost any financial question,” wrote the authors.
At the Fintech Sandbox Demo Day in April, Ye Tian, PhD., founder and CEO of Theia Insights, presented on using generative AI to develop thematic investing trends by drilling down into industries, sectors, and companies in a more accurate manner.
“Large language models (LLMs) synthesize vast amounts of information from the Internet, making content generation easy, but are not [always] reliable,” said Tian, a former Amazon Alexa scientist. “The challenge is that generative AI gives 90% of the answer and finance demands 100% accuracy,” said Tian.
According to Tian, Theia’s AI-based system is trained on a factual large language model (FLLM) for finance – which takes a large amount of numerical and language data from multiple sources, to generate clear content in language, tables, and graphs. The company is working on investing tools, factor-risk models, and analytics for individuals and institutions.
The startup developed its own LLM for finance and is distilling information into a so-called dynamic knowledge graph, said Tian. This kind of technology makes the LLM adaptable to up-to-date information and exposes emerging trends in response to news events, said Tian.
The firm started at the beginning of 2022 and launched its research platform In October. “It combines NLP with financial mathematics research. It is trustworthy; it does not hallucinate,” said its founder.
In terms of other use cases, some asset managers are currently using ChatGPT for code generation and code improvement. By entering an SQL query into ChatGPT, it completes, edits, and fixes bugs for the developer. This is a hyper-sophisticated version of GitHub’s Copilot, which can draw context and auto-complete code for a developer, noted Stauth.
But given the risk of leaking proprietary information to ChatGPT, some firms may not want to discuss their business problems with an AI bot, said Fidelity’s Stauth, who noted that some firms have locked down access to ChatGPT and are waiting for the enterprise-safe version from Microsoft.
ChatGPT for Traders
Apart from writing code, the financial industry is also starting to see applications for ChatGPT emerge for the institutional trading desk.
“Traders can benefit from AI and ChatGPT by breaking down the barriers between man and machine,” said FlexTrade EMEA’s Mahoney. For example, FlexTrade Systems has implemented ChatGPT in two aspects of its application suite, which is designed to revolutionize the way humans interact with data and trading systems, the company said.
In May, FlexTrade launched a prototype for AI-driven functionality within its multi-asset execution management system FlexTRADER EMS that uses ChatGPT, reported The Trade. The new solution, known as FlexA, (short for FlexAssistant) is a voice activated application developed by the firm’s in-house data science team. Using natural language prompts, “FlexA is a way of controlling the FlexTrade interface through voice or chat,” said Mahoney.
“By using plain spoken language, you don’t have to translate your thoughts into how a computer can understand it,” said Mahoney. “You can just express yourself clearly in English or another language and it will interpret that and convert it into computer-readable instructions,” he explained.
In a second instance, FlexTrade applied ChatGPT to implement a trade history query, so that a trader can search for trades within the EMS without the need for writing a complex query with heavy syntax, said Mahoney. For example, a buy-side user could tell ChatGPT to select all the small orders by average daily volume (ADV), rather than doing the task manually with clicking, scrolling and highlighting in a series of deterministic steps. “ChatGPT translates that query into API commands which we receive back from ChatGPT and run within our application,” he explained. In the future, Mahoney said, a trader could ask, what were my top broker algos in December by order count, and then right click to build an algo wheel with those brokers.
In fixed income, a buy-side trader might want to look at axe or list of bonds that a broker is buying or selling, or multiple disparate data sets, and it may be complex for every single order, he said.
However, financial firms are putting guardrails around their usage of ChatGPT to prevent sharing of confidential data. Mahoney said that the firm has taught ChatGPT what a broker means, what an order means, but it has not taught it how to press a button to execute a trade or send it to a broker.
“We have only told it how to do certain things that are menial tasks. There are no judgement calls in filtering or selecting rows or highlighting and calculating things. Judgement calls are where the trader adds their own value. The logic or the regulatory burden needs to stay with the human, which is to click the button, where the trader is accepting risk for these trades.
Many financial firms are waiting for an enterprise version of the OpenAI software to run the query on their own private servers. In FlexTrade’s case, the FlexA interface layer and trade query functions are in the prototype phase and not in production yet, said Mahoney.
“We are not comfortable with disclosing domain specific information to any third party. That is why everything is purely prototype,” said Mahoney. Before going into production with FlexA and trade query, Mahoney said he’s waiting for an API that can run on the firm’s own servers.
“We won’t be deploying it to production until we can have full control over what it does and not have that information leak,” says Mahoney.
Since generative AI is in its early stages, financial professionals are watching developments closely. OpenAI, which received a $10 billion investment from Microsoft and has been integrated into its Bing search engine, has spawned a host of rivals that are coming out with their own ChatGPT-like tools including Google and Amazon. “We are not wedded to any one tool yet,” said Mahoney.
Panelists on the Data Minds webinar cautioned that generative AI’s responses will be as good as the data it is trained on, and that the value will be in asking the right questions and keeping human oversight.
“At the end of the day, AI is not perfect and needs a lot of data and a lot of accurate and representative data,” said Fryer. “Having very representative data sets that are training your models, quality data sets and methodical human touch becomes important because we all worry about AI taking over the world. It’s going to be that human intervention that keeps it unbiased and helpful,” said Fryer.