Alt Data on the March with Machine Learning
January 24, 2018 | By: Ivy Schmerken
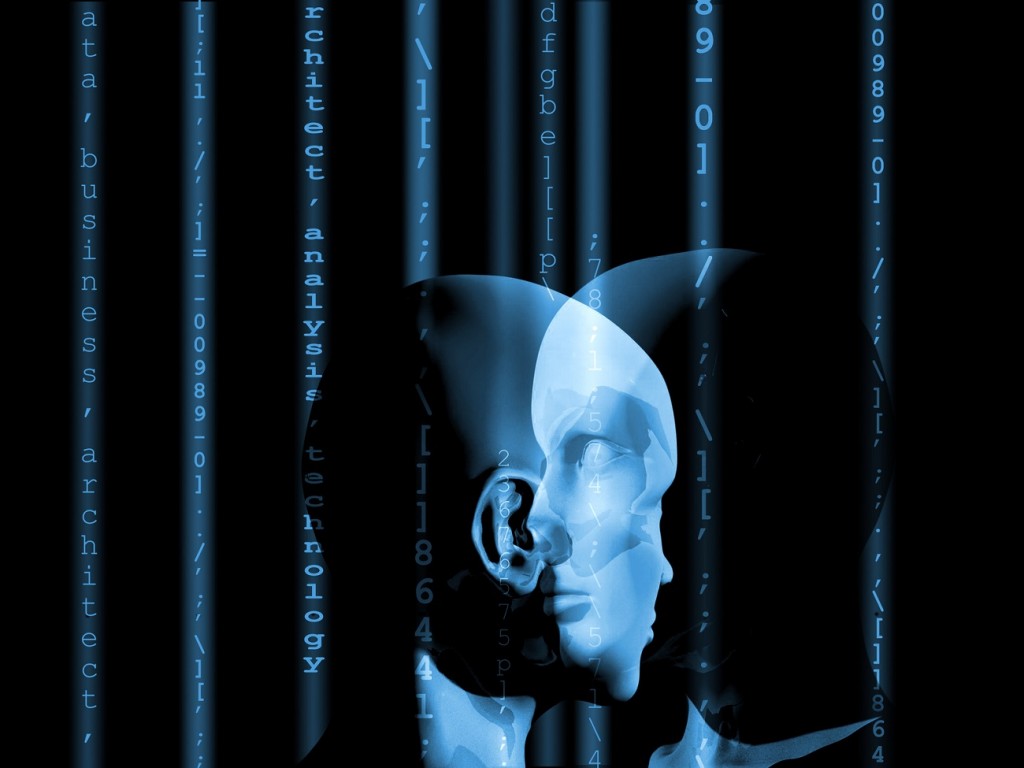
By Ivy Schmerken, Editorial Director
The explosion of alternative data sources, such as satellite images, sentiment analysis, and geolocation data, is having a profound impact on the field of quantitative investing.
Analyzing torrents of unstructured data requires sophisticated tools and technology, and this leads to opportunities as well as challenges.
Alongside the boom in alternative data, there is increasing demand for data scientists and machine-learning professionals who can work with petabytes of unstructured data sets. In January, Point72’s Aperio unit advertised for a “machine learning-data scientist” on AlternativeData.org, a public website that covers the industry.
And on Dec. 12, The Financial Times reported that AQR, a $208 billion hedge fund manager run by Clifford Asness, planned to explore big data. Despite previous doubts, AQR reportedly plans to experiment with machine learning “to parse through novel data sets such as satellite pictures of shadows cast by oil wells and tankers,” Asness told the FT.
Why is the growth in alternative data fueling the demand for data scientists with machine learning skills?
“It’s hard to make money on simple trades or macro-trades on basic relationships. So, everybody on the asset management side is chasing how to get that next edge,” said Mansi Singhal, cofounder at qplum, who spoke at TABB Forum’s Fintech Festival in November.
Based in Jersey City, NJ, qplum is a registered investment advisor that operates an online wealth management platform offering A.I. and machine learning-based portfolios of ETFs in stocks, bonds and real estate. The firm uses big data, algorithmic executions and risk parameters to run an automated end-to-end process. “Instead of asking people to write signals or strategies, the edge is to use a machine learning framework on it to extract features from data,” said Singhal in a follow-up interview.
Amidst the buzz surrounding alternative data, there are still concerns about the amount of resources, time and energy it takes to collect, process and reformat the data for use in algorithms and machine learning tools.
“Clearly, it’s not that simple. There are a lot of challenges in terms of collecting that data, coding that idea, and running the correct models,” said Patrick Pinschmidt, partner at Middlegame Ventures in Washington, D.C.
To extract value from alternative data, it requires an investment in infrastructure and talent, said qplum’s Singhal on the panel.
Data Scientists & Infrastructure
While traditional hedge funds will spend a lot of money on relationships and consultants, perhaps to obtain shipping or trucking data, qplum chose a different route. Firms can choose whether they want to spend money to get that data from a relationship or invest resources in developing their own data pipeline, continued Singhal. Investing in relationships or consultants is an ongoing expense, whereas once a data pipeline is built it can be utilized over and over again, she emphasized.
However, qplum’s Singhal said there has not been a better time to get one’s hands direct in the data space. For example, computational power is faster and infinite. In terms of data sources, “It’s like being a kid in a candy store,” she said.
While the term ‘alternative data’ is often associated with sentiment analysis from Twitter, Singhal said this is “very noisy data and hard to build sustainable models from.” Traditional asset managers “can start with stable, cleaner data,” she said.
Rather than pay consultants for data, qplum is downloading data from the Federal Reserve Economic Data – FRED – for free. FRED, which is provided by the Federal Reserve of St. Louis, offers access to 507,000 US and international time series from 87 sources. Anyone can scan the database for economic indicators, such as GDP, unemployment, and the consumer price index.
Though the FRED data is free, engineers and application programming interfaces (APIs) are needed to process that data, said Singhal. “To me the biggest challenge is processing — being able to build that pipeline. The actual code to derive the alpha part is so much smaller than the whole wrapper around it which is focused on cleaning and processing, reconciliation and post-trade processes.”
But she added, “It’s not easy to set up, unfortunately.”
Alt Data Ecosystem
However, firms no longer have to do all the work on their own. An entire ecosystem of alternative data providers, domain experts, data platform providers and visualization tools has evolved.
According to Alternativedata.org, there are 213 alternative data providers, and the alt data industry is expected to be worth $350 million in 2020.
On the sell side, there are many use cases, as well.
At TABB’s A.I. /alt data panel, Nikhil Singhvi, global head of market and client connectivity at Credit Suisse, said use cases on the sell side include client acquisition, research, trading operations and compliance. “Each of these uses cases has disparate data sets, and are at different levels of maturity,” he said.
One of the challenges is that models developed from alternative data would need more testing before releasing them to clients, said Singhvi.
In the old days, quants sat next to proprietary traders. “You could come up with a model and work with the prop trader to be able to really test that strategy or test that concept,” said Singhvi. Now, the prop traders are no longer there, [as a result of the Dodd Frank Volcker Rule], and this is a challenge, he said.
While there is a lot of work being done to use the alternative data sets on Wall Street, Credit Suisse’s Singhvi said he doesn’t think it’s going to override everything else that you see from the accounting statements. “I don’t see that happening anytime soon,” he said. “If it’s another data point, then we look at it and build in other data elements around it and improve the quality of research,” he said.
Even if usage of alternative data by Wall Street is in its early stages, panelists suggested that a fundamental transformation of quant methods is occurring. While people on the sell side have been doing quantitative analytics for a long time, Singhvi said that alternative data is “changing their thinking from a statistic-based quant to AI and machine learning. It’s a big change,” he said.
Past blog posts about Alt Data issues: