Data Dominates Buy-Side Approach to FX Trading
June 10, 2021 | By: Ivy Schmerken
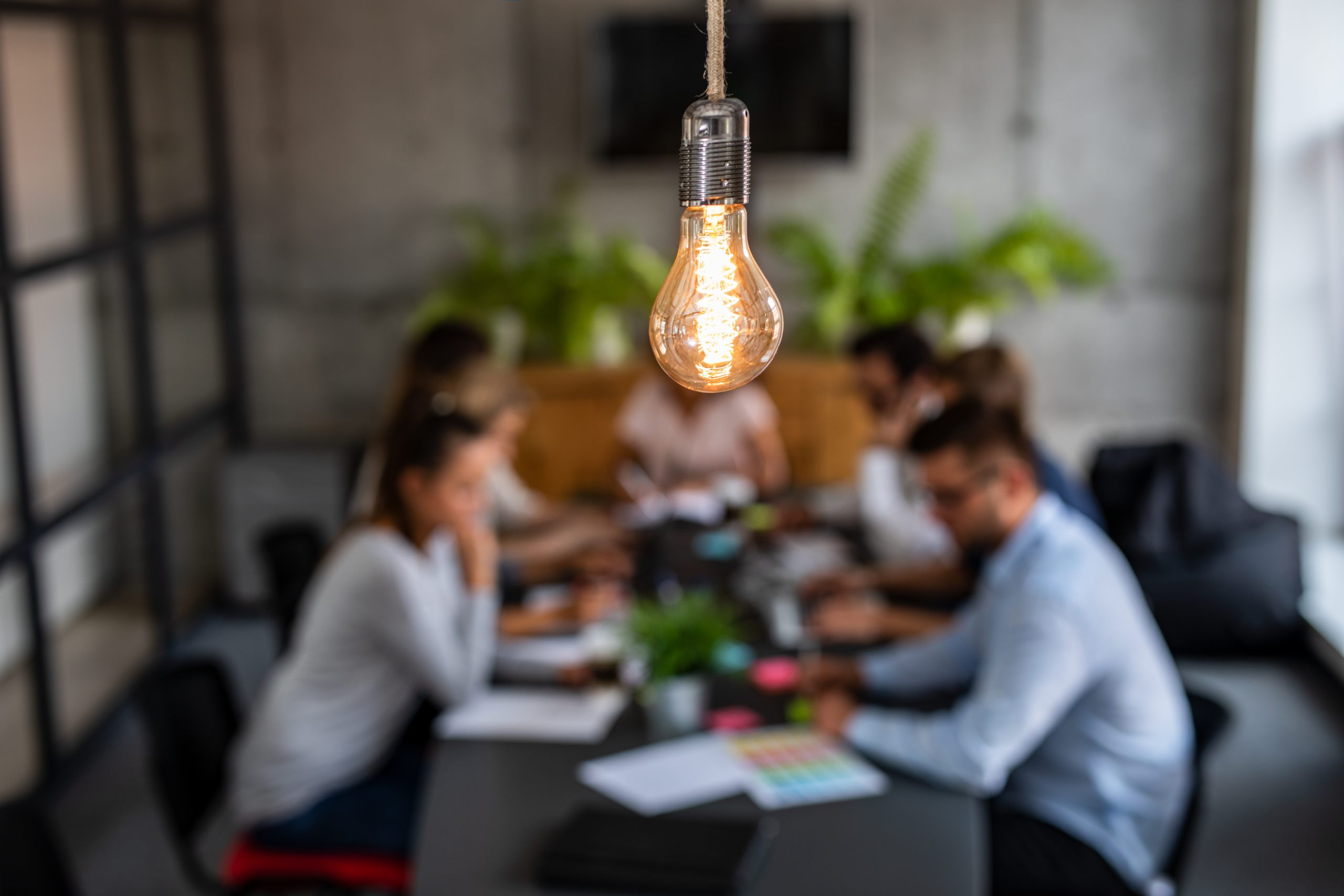
Senior buy-side traders offered differing views on the definition of high-touch and low-touch trading in foreign exchange (FX) at a roundtable in May, shedding light on efforts to rank their brokers and incorporate data analytics into their decisions.
Discussing their usage of FX electronic trading protocols and algorithms for “chunkier trades,” buy-side heads of FX and derivatives trading desks, shared their views on a roundtable in May moderated by The Hive FX Network and co-hosted by FlexTrade Systems.
With liquidity provided by banks, dealers, and venues, buy-side FX participants explained how they rely on pre-trade analytics and either internal or external transaction cost analysis (TCA) services to benchmark their trades and evaluate pricing from their brokers.
Deciding whether to go low-touch or high-touch is predominantly driven by the individual currency pair, size of order, time of day, and whether the currency is deliverable or a non-deliverable forward (NDF), or whether a trade can go through algos, according to several buy-side FX traders who represented large investment management firms.
Though high-touch trading will always exist for block trades, several FX buy-side traders said they are taking steps to bring more automation into their workflows. Large asset managers are inundated with hundreds upon hundreds of tickets from mutual funds and segregated mandates – the majority of which are in liquid currency pairs.
“The majority of our flow is through the RFQ (request for quote,) so it’s really trying to automate that flow first and then looking at algos,” said an FX roundtable participant.
However, the definition of low-touch versus high-touch trading varied across the spectrum of investment shops based on the level of internal analytics, technology, and views on automation.
One sophisticated large asset manager defined low-touch trading as using request-for-quote (RFQ) to receive firm prices from liquidity providers, whereas anything that a trader had to touch or exercise discretion on – such as an algorithm – was considered high touch.
Traders also consider the portfolio manager’s sensitivities when deciding how to execute. “We do have certain patterns that they insist on having their trades executed by a trader and don’t want to use algos,” said a roundtable participant.
Another firm built an internal “RFQ wheel,” an analytical tool to help decide where to go with their trades. A participant on the equity and FX side, said it was up to the traders’ discretion whether to execute electronically or use RFQs. In another firm’s case, most FX trades were low-touch and handled through either an internal messaging bus infrastructure or algos, while most NDFs required high-touch.
Challenges with Pre-Trade & TCA
Demand for data particularly for pre-trade analysis in FX was a key theme, with many on the buy side using internal analysis to divide trades into different buckets by price, size, and currency pair, then ranking their brokers’ performance. For example, one firm had an advanced analytics team building an internal liquidity monitor taking in external data sources and historical quotes.
Data-driven decision making was seen as key to removing the buy-side trader’s bias in sending flow to brokers. “We’re really working hard to get data as close to the point of execution as we can,” said a member of a TCA team at a large asset manager.
But a Greenwich Associates study on algorithmic trading published in February found that less than half of the buy side is using TCA benchmarks, “and even fewer use the sophisticated TCA systems that have served as an accelerant for algos in other asset classes,” according to a summary of the report.
Although pre-trade cost data and TCA are available from dealers, this is often inefficient because the buy side needs to access it on multiple single-dealer platforms. The hassle of logging into different bank pre-trade platforms resulted in one buy-side firm infrequently using this data.
Firms also said they were struggling with how to integrate pre-trade and post-trade into their processes. With so many algos in FX, it is “difficult to gauge the true pre-trade cost model,” and there are “big deviations across the different bank platforms,” according to a buy-side FX participant.
One of the hurdles for FX TCA is the lack of trade disclosure in FX and this means FX market participants do not have access to a consolidated, universal data on pricing and execution that feed algorithms in equities, and hence make it easier to benchmark trades, noted Greenwich.
“The FX market has fragmented liquidity, so it is difficult to use equity-market oriented measurement tools for TCA, which requires comparing apples with apples,” commented Howard Tai, principal at Frontline Global Markets LLC, an expert on buy-side foreign exchange and equity derivatives risk management topics, in an interview.
“The whole concept of a central limit-order book doesn’t exist in FX market structure,” said Tai, adding “It’s a series of large and small pools fragmented everywhere.” The pricing is not uniform because the FX market is based on bilateral trading where the prices depend on the buy-side relationship with the banks, dealers, and other liquidity providers, Tai noted. “Price depends on your volume, your credit worthiness, and what other parts of your firm does business with their firm that touches each other. That is all part of the relationship,” said Tai.
On the algo front, Tai said that “some of the heaviest volumes of algo usage by large buy-side firms are conducted in in single-dealer platforms because of their relationships over many years with the money-center global banks.” From a TCA benchmark standpoint, they are not spreading their liquidity out to different environments like multi-dealer platforms and ECNs. “The disadvantage is they don’t see the aggregated market,” he said. If they are using a volume benchmark, it is limited to the volume they are seeing in the single dealer pools.
Classifying FX Trades
During the roundtable, one FX buy-side trader explained how the desk grabs the historical quotes via request-for-stream feeds – “both winners and losers” — from two-sided markets — and transfers them to an internal database. Next, it breaks each trade into three components – spot trades, spot risk, and forward points risk under a week. Classifying trades into different buckets, it analyzes spreads that dealers have shown to the firm, and creates tiers for its brokers by currency pair, by trade type, and other metrics. In order to randomize the broker selection process, the buy side can pick a certain number of dealers from each bucket. “So, if you have a higher tier, you have a higher chance of seeing a trade,” said an FX buy-side trader.
The buy side’s method of ranking brokers could control the odds of their seeing trades based on historical performance. Reviewing trades executed six months ago could penalize a broker for past performance or liquidity conditions, whereas looking at the more recent period of three months ago would acknowledge their improvement.
Providing more targeted feedback to the sell-side has resulted in tighter spreads, according to the buy side. Instead of being priced in a generic way, “there are discrete ways in which the dealers can adjust pricing whether it be by currency, or size, or combination of factors,” said a roundtable participant. In turn, dealers get feedback on their precise percentile rank and how many trades they are seeing.
Automation: Varying Definitions
While FX traders spoke about automating their smaller trades, it was unclear how they defined automation. For example, one trader said she was sending RFQs out by currency pair but is looking to apply automation rules for low-touch orders so that RFQs could be sent out automatically.
Another trader used an auto-ex capability via FX Connect for small orders in the most liquid currency pairs such as euro-yen or euro-sterling, sending them all at once, but bigger trades outside of G-3 currencies such as Mexican dollar or Turkish lira are sent through algos. In the latter case, the firm has no systematic or quantitative way of determining what is the best choice of broker algo other than go on “gut feel” or what they did in the past.
Given that each trader has their own biases, manually selected brokers is not regarded as a value add. “If a trader goes in and selects five brokers for a 3 million Eurodollar, (based on who they think is good or bad), that is not a value-add,” said one buy-side trader.
The goal is to turn those smaller trades into one-touch executions which can be executed as a basket trade to free up time to focus on larger blocks or answer a portfolio manager’s questions, said the same buy-side trader.
The biggest takeaway from the roundtable is that data is critical to deciding how and where to execute FX trades, and this includes both internal and external data sets from third-party providers and peer-data from other buy sides.
“It’s more about using systematic assessment of spreads and hit ratios so that we can create a tiering of our banks, essentially using data to eliminate bias and provide feedback to banks, and ultimately to inform our future execution decisions,” said a roundtable participant.
Focusing on the next 18 months, several buy-side FX traders talked about the potential for algo-wheels in FX to help quantify their choice of broker algo and incorporating “peer data” from other buy-side firms into their TCA. But the ability to integrate these data points into a common technology framework is still a work in progress.