Enhance Institutional Trading Performance: Leveraging AlgoWheels and Advanced Cost Models
In global equities trading, institutional participants invest substantial effort in selecting the best mix of brokers, algorithms, and strategies to reduce trading costs and deliver best execution. At times, this requires painstakingly stitching together and normalizing disparate data sources and parameters across brokers. How can firms leverage AlgoWheels, advanced cost models, and TCA to run comparisons that identify optimal brokers?
"*" indicates required fields
What to Expect
This paper explores how firms can leverage FlexTrade’s AlgoWheel and TCA & Analytics tools to enhance institutional trading performance. It tackles topics such as obstacles in evaluating algo performance, integrating pre-trade and post-trade cost models with peer data in the AlgoWheel, and reweighting the AlgoWheel using a reinforcement learning based feedback loop.
Section I Key Obstacles in Evaluation of Algo Performance
Section II Integrating Pre-Trade and Post-Trade Cost Models with Peer Data in the AlgoWheel
Section III Applying Pre-Trade Cost Models to AlgoWheel
Section IV Reinforcement Learning-Based Feedback Loop
Key Take Aways
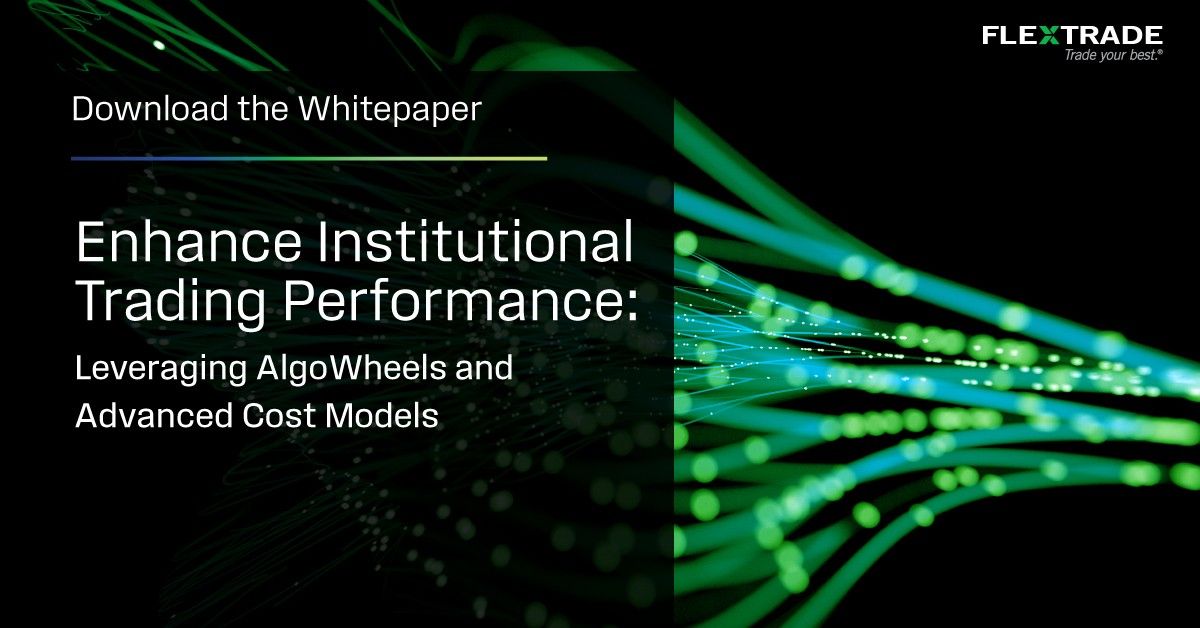
Key Findings
- Pre-trade cost models address major obstacles in evaluating algo performance, reducing market noise and adjusting for order difficulty.
- Normalized parameters assist in conducting proper experiment design and comparison. FlexTrade’s post-trade model within the TCA & Analytics tool adjusts for order difficulty allowing institutions to run comparisons that are insightful and actionable.
- The application of the post-trade model to the feedback loop resulted in a 60% reduction of the time it took to identify the optimal broker.